FlashBlade//EXA From Pure Storage Has Arrived – The Data Storage Platform to Transform Large-scale AI and HPC.
The announce of FlashBlade//EXA has been received with much market excitement - this innovative and cutting-edge solution offers the next generation of massive storage throughput, for the most demanding AI workloads.
Being the newest member of the FlashBlade family and optimised for AI workloads with ultra scaleability and extreme levels of throughput, read on to learn more how this technology can make your organisation's AI ambitions become reality...
What do high-performance car manufacturers and Pure Storage have in common? Just as top-tier automakers design cutting-edge race car models that push the limits of speed and efficiency, FlashBlade//EXA is built to deliver next-level performance for AI and HPC workloads. FlashBlade//EXA is similar to those examples in that it is a FlashBlade® system with Purity//FB highly optimized to provide next-level performance for the most demanding AI workloads. It complements the high-performance FlashBlade//S™ and high-density FlashBlade//E™ models that have been proven in the enterprise market and recognized in the Gartner® Magic Quadrant™ for File and Object Storage Platforms as a leader for four years in a row.
The Business Challenge of Rapid AI Advancements
As AI innovation expands, many businesses are quickly discovering value in augmenting or revolutionizing their existing operations with model training and inference. This accelerated growth has increased the adoption of AI workflows across pre-processing, training, testing, fine-tuning, and deployment—each benefiting from more powerful GPUs and larger, multimodal data sets.
This expansion has also introduced new infrastructure challenges. Legacy storage scalability, checkpointing, management, and metadata performance limitations at scale are creating bottlenecks and hindering the full utilization of expensive GPU-oriented infrastructures and slowing progress and innovation. This greatly impacts AI’s aggressive ROI financial pressures—any infrastructure dedicated to it has to run at peak performance to ensure the fastest time to value possible for model training and inference. Lost time is lost money.
Business Challenges Are Amplified with Large-scale AI Workflows
This business challenge of idle GPUs equating to lost time and money is exponentially amplified at scale, e.g., GPU cloud providers and AI labs, for two reasons. First, massive operational efficiency at scale is core to their profitability and ranges well beyond what most on-prem/in-house data centre operations manage. Second, service providers subscribe to the core tenet of avoiding any resources running idle. For them, idle GPUs in any AI model are a lost revenue opportunity—storage inefficiencies at their level of operations can be damaging.
Traditional high-performance storage architectures have been built on parallel file systems and were designed and optimized for traditional, dedicated high-performance computing (HPC) environments. HPC workloads are predictable, so the parallel storage systems could be optimized for specific performance scaling. Large-scale AI-based workflows and models are different from traditional HPC because they are more complex, involving many more parameters that are also multimodal by including text files, images, videos, and more - all of which need to be processed simultaneously by tens of thousands of GPUs. These new dynamics are quickly proving how traditional HPC-based storage approaches struggle to perform at a larger scale. More specifically, the performance of traditional parallel storage systems becomes contentious with servicing metadata and associated data from the same storage controller plane.
This emerging bottleneck requires new thinking for metadata management and data access optimizations to efficiently manage diverse data types and high concurrency of AI workloads at a service provider scale.
Extreme Storage Scaling Requirements with AI Workload Evolution
As data volumes surge, metadata management becomes a critical bottleneck. Legacy storage struggles to scale metadata efficiently, leading to latency and performance degradation—especially for AI and HPC workloads that demand extreme parallelism. Traditional architectures, built for sequential access, can’t keep up. They often suffer from rigidity and complexity, limiting scalability. Overcoming these challenges requires a metadata-first architecture that scales seamlessly, supports massive parallelism, and eliminates bottlenecks. As the AI and HPC opportunity evolves, the challenges are only compounded.
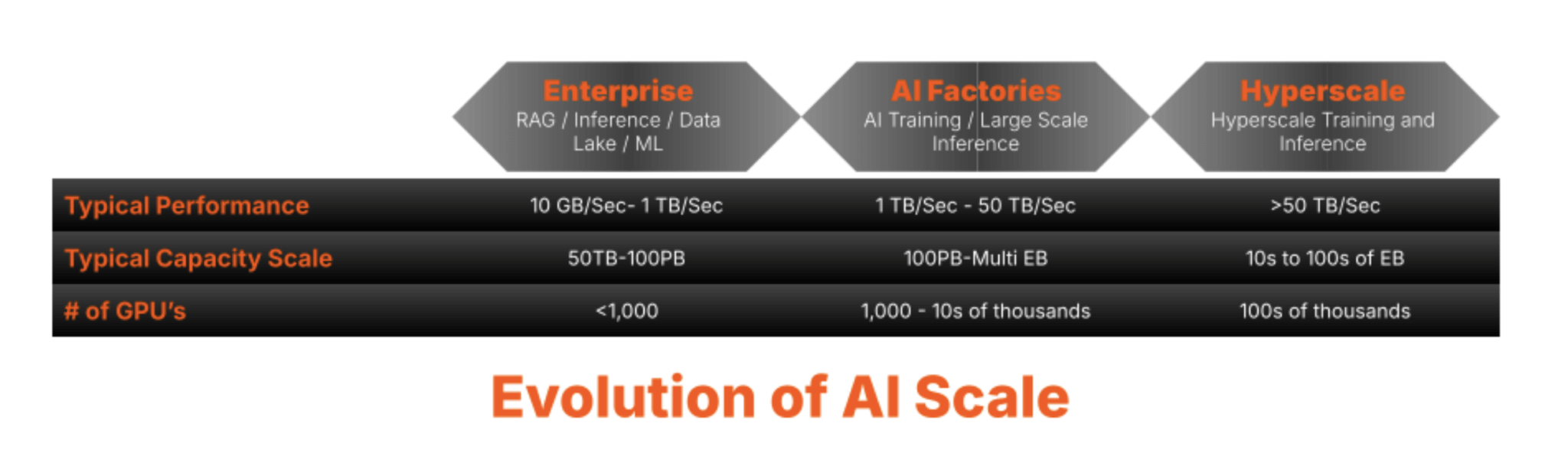
The proven metadata core available in FlashBlade//S has helped enterprise customers address demanding AI training, tuning, and inference requirements by overcoming metadata challenges, such as:
- Concurrency management: Handling massive volumes of metadata requests across multiple nodes efficiently
- Hotspot prevention: Avoiding single metadata server bottlenecks that can degrade performance and require ongoing tuning and optimizations
- Consistency at scale: Ensuring synchronization across distributed metadata copies
- Efficient hierarchy management: Optimizing complex file system operations while maintaining performance
- Scalability and resiliency: Sustaining high performance as data volumes grow exponentially
- Operational efficiency: Ensuring that management and overhead is minimized and automated to support efficiency at scale
FlashBlade//EXA Addresses AI Performance Challenges at Scale
Pure Storage has a proven track record of supporting customers across a broad range of high performance use cases and at every stage of their AI journey. Since introducing AIRI® (AI-ready infrastructure) in 2018, they continued to lead with innovations like certifications for NVIDIA DGX SuperPOD™ and NVIDIA DGX BasePOD™ as well as turnkey solutions like GenAI Pods. FlashBlade has earned trust in the enterprise AI and HPC market, helping organizations like Meta scale its AI workloads efficiently. Pure's metadata core is built on a massively distributed transactional database, and key-value store technology has ensured high metadata availability and efficient scaling. By applying insights from hyperscalers and leveraging advanced metadata core proven with FlashBlade//S, Pure Storage has the unique ability to deliver extreme performance storage that overcomes the metadata challenges of large-scale AI and HPC.
FlashArray//C20: Platform Capabilities Optimised for Edge Deployments and Smaller Workloads
An extension of the existing FlashArray™ architecture, FlashArray//C20 offers enterprise-class performance, reliability, and agility at capacities and prices that are more accessible for edge use cases and smaller deployments. It brings all the power and capability of the Pure Storage platform and the FlashArray//C family to a model that’s specifically sized and priced to support lower-capacity deployments. And unlike other “entry-level” systems, FlashArray//C20 can non-disruptively upgrade to higher models in the FlashArray//C family and next-gen controllers to grow as you need in the future. This is a unique benefit of the Pure Storage platform that is unmatched by any other vendor in the industry.
Companies that are considering moving to all-flash for their business-critical workloads can now get the simplicity and power of the Pure Storage platform at a much lower capacity and the same competitive £/TiB, making it an attractive way to move off hybrid systems.
Customers who are already harnessing the capabilities of the Pure Storage platform can now deploy FlashArray with smaller capacities across use cases while leveraging the same simple experience.
Enter FlashBlade//EXA
As extreme end-to-end AI workflows push the boundaries of infrastructure, the need for a data storage platform that matches this scale has never been greater. FlashBlade//EXA extends the FlashBlade family, ensuring large-scale AI and HPC environments are no longer constrained by legacy storage limitations.
FlashBlade//EXA is designed for AI factories and delivers a massively parallel processing architecture that disaggregates data and metadata, eliminating bottlenecks and complexity associated with legacy parallel file systems. Built on the proven strengths of FlashBlade and powered by Purity//FB’s advanced metadata architecture, it provides unmatched throughput, scalability, and simplicity at any scale.
Whether supporting AI natives, tech titans, AI-driven enterprises, GPU-powered cloud providers, HPC labs, or research centers, FlashBlade//EXA meets the demands of the most data-intensive environments. Its next-generation design enables seamless production, inference, and training—offering a comprehensive data storage platform for even the most demanding AI workloads.
Pure's innovative approach in how they modified Purity//FB, which involved splitting the high-speed throughput network-based I/O into two discrete elements:
- The FlashBlade array stores and manages the metadata with its industry-leading scale-out distributed key/value database.
- A cluster of third-party data nodes is where the blocks of data are stored and accessed at very high speed from the GPU cluster over Remote Direct Memory Access (RDMA) using industry-standard networking protocols.
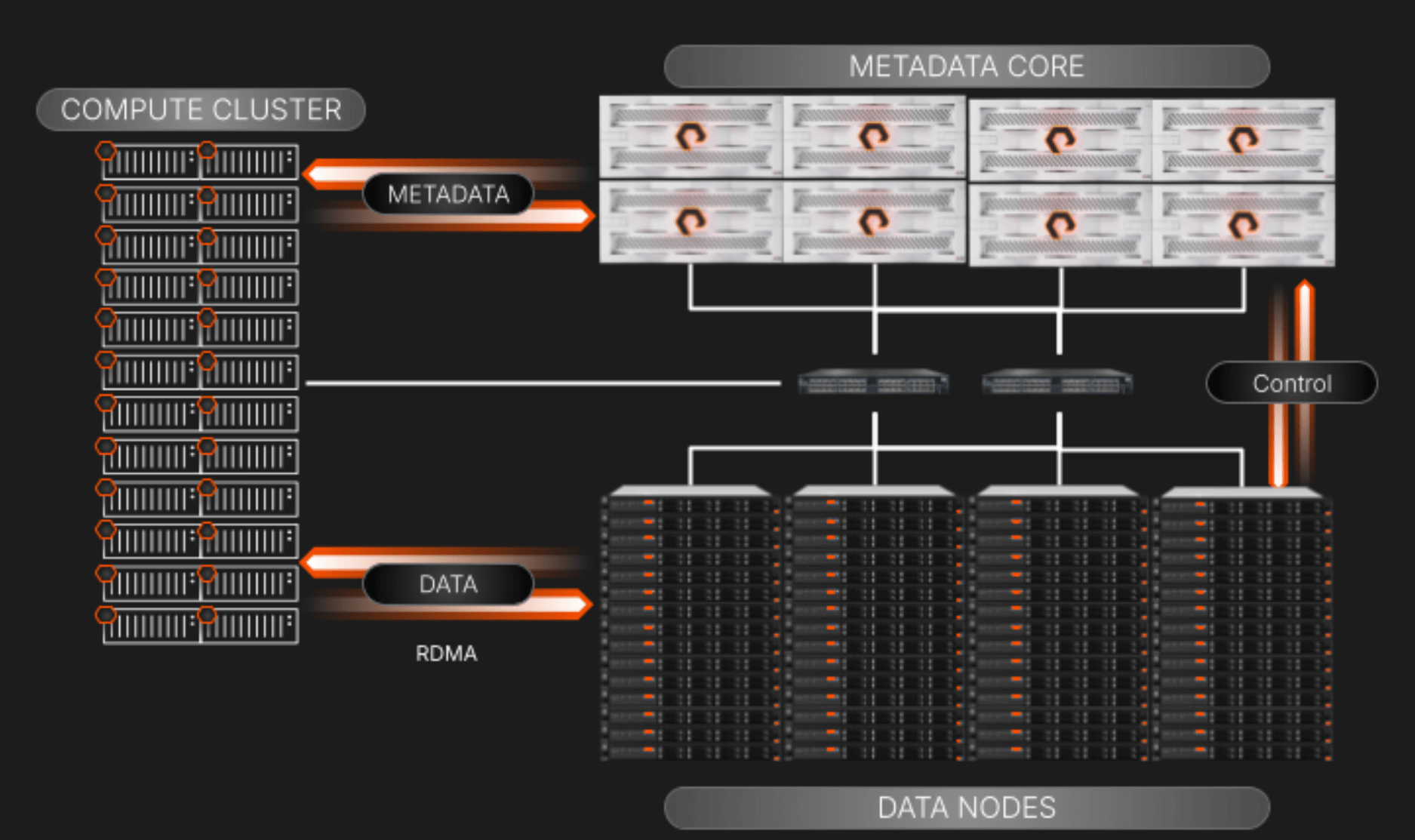
This segregation provides non-blocking data access that increases exponentially in high-performance computing scenarios where the metadata requests can equal, if not outnumber, data I/O operations.
Why Off-The-Shelf Servers and SSDs for Data Nodes?
Large-scale AI environments can have an established investment of 1U and 2U servers with SSDs as infrastructure building blocks. FlashBlade//EXA leverages off-the-shelf servers for the data plane—making it easier to fit into the target customer’s architecture (in this case, large-scale environments). This highlights an important point about the data storage platform:
Purity’s strength, as the heart of the platform, lies in its ability to be modified to address new use cases, even if it means stretching to operate outside Pure's own hardware. Solving challenges with the software is a core principle because it’s a more elegant approach and provides a faster time to value for customers.
These off-the-shelf data nodes give customers the flexibility to adapt over time and can be driven by the customers’ evolution on how they leverage NAND flash in their data centers.
A High-level View of FlashBlade//EXA Components and I/O
While separating the metadata and data servicing planes out, we’ve focused on keeping the elements in the above diagram simple to scale and manage:
- Metadata Core: This services all metadata queries from the compute cluster. When a query is serviced, the requesting compute node will be directed to the specific data node to do its work. The array also oversees the relationship of data nodes to metadata via a control plane connection that is behind the scenes on its own network segment.
- Third-party data nodes: These are standard off-the-shelf servers to ensure broad compatibility and flexibility. The data blocks reside on the NVMe drives in these servers. They will run a “thin” Linux-based OS and kernel with volume management and RDMA target services that are customised to work with metadata residing on the FlashBlade//EXA array. Pure will include an Ansible playbook to manage deployment and upgrades to the nodes to eliminate any concerns about complexity at scale.
- Parallel access to data using the existing networking environment: FlashBlade//EXA employs an elegant approach that leverages a highly available, single-core network utilizing BGP to route and manage traffic among metadata, data, and workload clients. This design enables seamless integration into existing customer networks, simplifying the deployment of highly parallel storage environments. Importantly, all leveraged network protocols are industry standard; the communication stack contains no proprietary elements.
Unpacking the Challenges of Legacy High-performance Storage with Parallel File Systems and Disaggregated Models
Many storage vendors targeting the high-performance nature of large AI workloads only solve for half of the parallelism problem -offering the widest networking bandwidth possible for clients to get to data targets. They don’t address how metadata and data are serviced at massive throughput, which is where the bottlenecks at large scale emerge. This makes sense since the intent of NFS’s design when Sun Microsystems created it back in 1984 was to simply bridge the gap between local and remote file access, where the design focus was functionality over speed.
Challenges of Scaling with Legacy NAS
The design and scale of legacy NAS prohibits supporting parallel storage because of its single-purpose design to service operational file shares and inability to scale I/O linearly as more controllers are added.
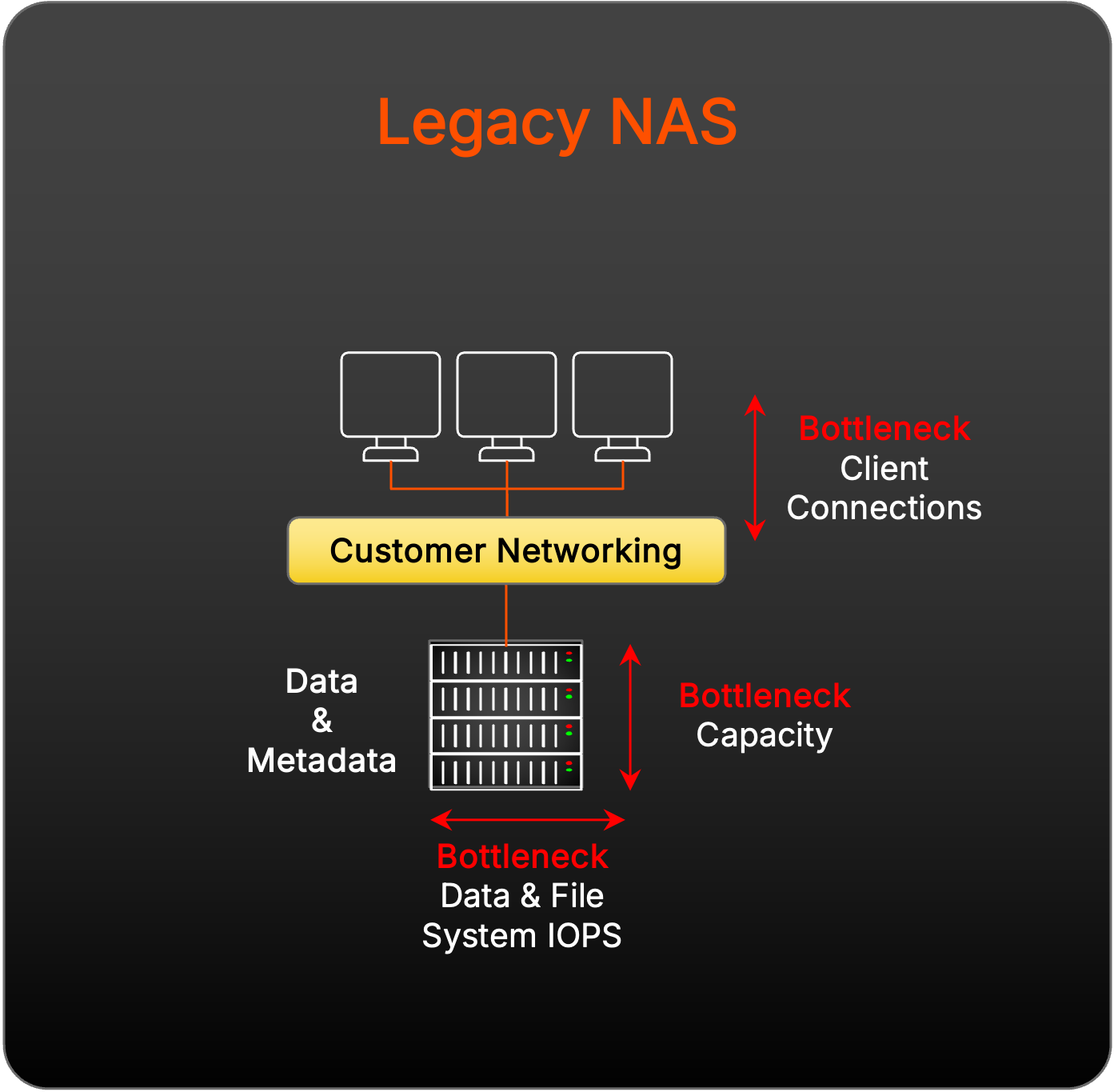
Challenges of Scaling with Traditional Parallel File Systems
Even before the current rise of AI, some legacy storage providers leveraged specialised parallel file systems like Lustre to deliver high-throughput parallelism for high-performance computing needs. While this worked for several large and small environments, it is prone to metadata latency, extremely complicated networking, and management complexity, often relegated to PhDs who oversaw their HPC architectures and associated soft costs when scaling to larger needs.
Challenges of Disaggregated Data and Compute Solutions
Other storage vendors have architected their solutions to not only rely on a purpose-built parallel file system but also add a compute aggregation layer between workload clients and the metadata and data targets:
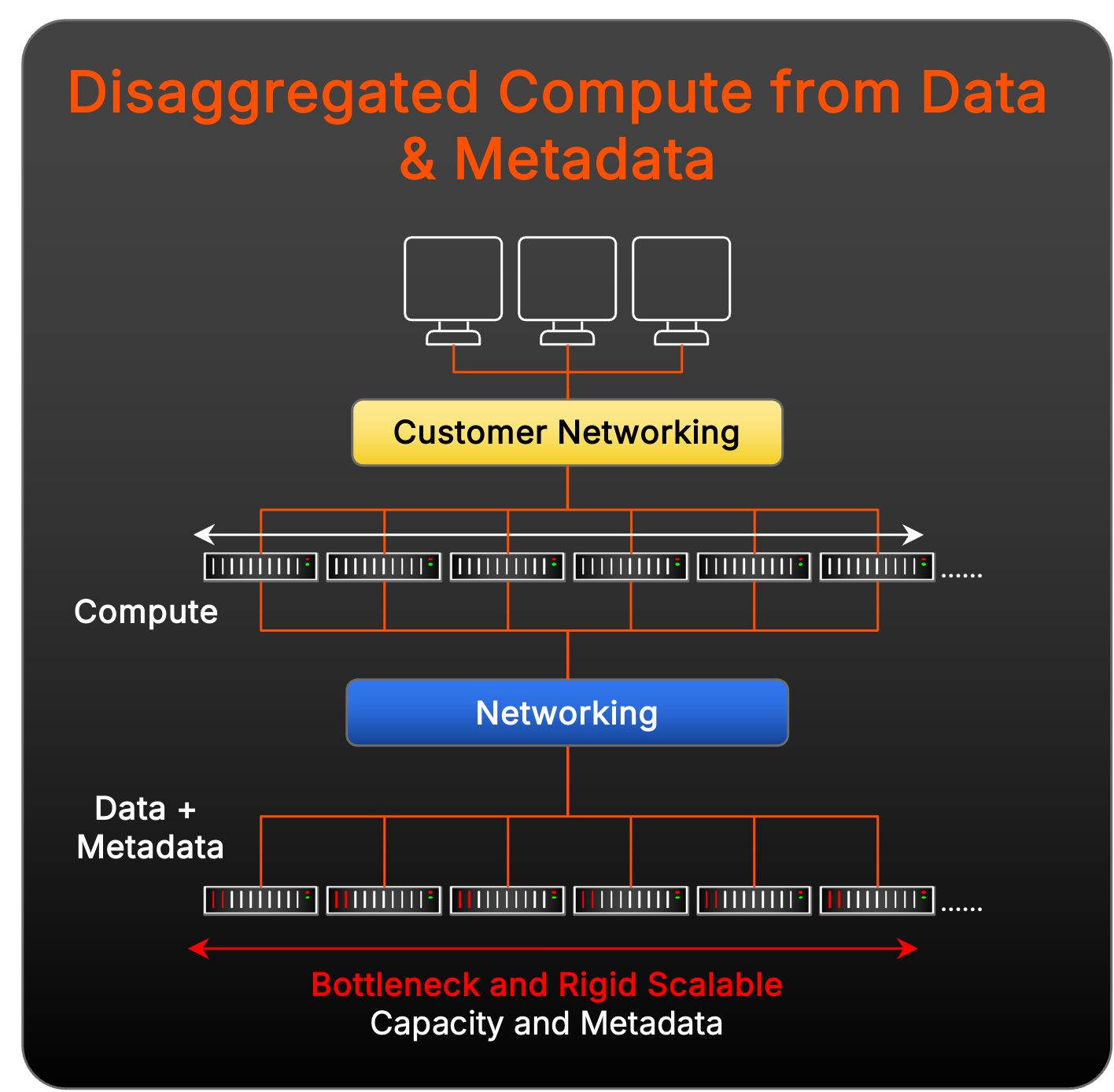
This model suffers from expansion rigidity and more management complexity challenges than pNFS when scaling for massive performance because it involves adding more moving parts with compute aggregation nodes. There is another potential challenge -the deployment of disaggregated data access functions in this model risks unexpected latency in the stack because its networking becomes much more complicated in managing the addressing, cabling, and connectivity with three discrete layers versus what is needed for pNFS.
Additionally, each data and metadata node is assigned a fixed amount of cache where metadata is always stored. This rigidity forces data and metadata to scale in lockstep, creating inefficiencies for multimodal and dynamic workloads. And, as workload demands shift, this linear scaling approach can lead to performance bottlenecks and unnecessary infrastructure overprovisioning, further complicating resource management and limiting flexibility.
This FlashBlade//EXA announcement revolutionises performance, scalability, and simplicity for large-scale AI workloads. This is jusy the beginning of your journey with Pure Storage - If you require assistance on the above, please contact your COOLSPIRiT Account Management Team directly for information on the next steps to take. Alternatively, call 01246 454 222 or email hello@coolspirit.co.uk.